Will Robots Steal Your Job?
Scientists are approaching “the end of insight.” Can computers replace them, too?
Can robots work as scientists? At first, this seems like a silly question. Computers are pervasive in science, and if you walk into a large university lab today, there’s a good chance you’ll find a fully fledged robot working alongside the lab-coat-wearing humans. Robots fill test tubes, make DNA microarrays, participate in archaeological digs, and survey the oceans. There are entire branches of science—climate modeling and genomics, for example—that wouldn’t exist without powerful microprocessors. Machines even play an integral part in abstract fields of discovery. In experimental mathematics, humans rely on computers to inspire new lines of thinking and investigate hypotheses. In 1976, mathematicians used computers to prove the four-color theorem, and machines have since been used in several other proofs.
Still, in most scientific fields, there’s a clear division of labor between humans and computers. Machines occupy themselves with gruntwork—they do the calculating, graphing, mixing, filling, watching, and waiting. Wherever there’s work that’s too tedious, time-consuming, or boring for a human—even a graduate student—you’ll find a robot ready to help. Humans save themselves for that most rarefied pastime: thinking.
In the last section, I wrote about lawyers who believe their thinking-heavy profession is beyond the scope of machines; in science, that feeling is so pervasive as to be unquestioned. Here, people do everything that’s remotely interesting—they come up with theories, design creative experiments, and dream up deep questions for the machines to help answer. So far, this division of labor has worked out pretty well. The humans are happy to give up the dirty work—we’ll even go so far as to concede that the machines are really good at all that tedium, and that without them we wouldn’t be anywhere. The machines, meanwhile, appear to have little capacity to match humans when it comes to mental heavy lifting. All seems well: The humans are in charge, the robots are content, and science progresses.
Then, two years ago, Hod Lipson and Michael Schmidt announced the first stirrings of robotic thinking. Lipson, a computer science professor at Cornell, and Schmidt, then a graduate student in Lipson’s lab, created a computer program that, given a raft of data from physical systems, can describe the natural laws that apply to that system. When they fed their software the motion-capture coordinates of a swinging double pendulum, the machine pondered the data for a couple days, then spat out the Hamiltonian equation describing the motion of such a system—an equation that represents the physical law known as conservation of energy. Their software needed no prior knowledge to discover this law. It wasn’t familiar with gravity, energy, geometry, or anything else. It simply did what human scientists have done since the time of Newton. It looked at the world, came up with theories about how it works, tested them, and then produced a law.
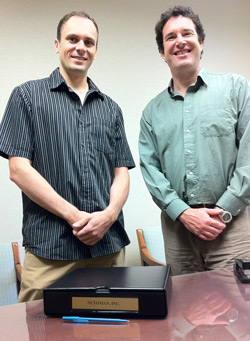
Image is courtesy of Schmidt.
Lipson and Schmidt called their program Eureqa, and they made it available for free on the Web. It has since yielded several new discoveries in a range of fields, discovering scientific laws that we’d never known. Lipson and Schmidt recently worked with Gurol Suel, a molecular biophysicist at the University of Texas Southwestern Medical Center, to look at the dynamics of a bacterium cell. Given data about several different biological functions within the cell, the computer did something mind-blowing. “We found this really beautiful, elegant equation that described how the cell worked, and that tended to hold true over all of our new experiments,” Schmidt says. There was only one problem: The humans had no idea why the equation worked, or what underlying scientific principle it suggested. It was, Schmidt says, as if they’d consulted an oracle.
This should terrify scientists. If robots can now outsmart us, what’s left for people to do? More importantly, if we’re entering an age in which machines will be the primary discoverers of new scientific wisdom, what will that mean for human knowledge? We may be able to make use of the laws and equations uncovered by computers, but it’s quite possible that some of it will be too complex for even the smartest humans to understand.
In other parts of this series, I’ve touched on how robots will help many of us—we’ll get better medicine and better legal services—while putting others on the unemployment line. Thinking machines like Eureqa present a knottier problem. Over the long run, they’ll likely put human scientists out of work. And they may even help change the world in positive ways—Schmidt argues that a machine like Eureqa could determine the strongest, lightest metal alloys to use in industrial applications. At the same time, though, scientific thinking machines might leave us utterly confused about how the world works. We’ll just have to take the computers at their word.
At the moment, Schmidt and Lipson’s machine is still very much subservient to humans—it depends on people to feed it data, and to direct it toward novel research problems. Eureqa is quite simple in design. After it’s fed data about a particular process (the swinging of a pendulum, the dynamics of a cell), the computer generates a huge field of potential equations. These initial equations are random, and the vast majority of them will not apply. But a few of these random equations will show some agreement with the physical world. “We take the ones that are slightly better than the others, and we randomly recombine them to get new equations—and then we repeat the process over and over again, billions and billions and billions of times, until we’ve exhausted the space of short, simple equations,” Schmidt says. In the end, this Darwinian process tends to come up with equations that describe “invariant relationships”—that is, equations that apply across all the data. Such invariant relationships are often associated with fundamental laws of nature: the conservation of energy, Newton’s laws of motion, the mass-energy equivalence.
Both Lipson and Schmidt stress that even if a system like Eureqa becomes the standard way scientists discover natural laws, human scientists will still need to determine what the computer’s formulas mean. “This gets into a deeply philosophical area, which is, What is insight?” Lipson says. “I think it’s a subjective thing—insight and meaning represent some kind of feeling, some kind of sense that you really understand what’s going on. You’ll still need humans for that.”
But there are two problems for human scientists expecting to find long-term employment as meaning-finders. First, Lipson is already working on ways for the computer to explain itself—that is, to describe, in terms that humans might understand, what its equations mean. For the pendulum, we might explain to the computer that we understand a certain quantity as representing energy. Then the software will have to explain its new finding using only the concepts that we’ve taught it. “It’s a little bit like if a child asks you, ‘What’s an airplane?’ and you say, ‘Well, it has wings like a bird, and it has an engine, like a car.’ ” At some point, though, the computer might discover laws that are impossible for us to understand. “It would be like trying to explain Shakespeare to a dog,” Lipson says.
Steven Strogatz, a mathematician at Cornell, coined a name for this problem in an essay he wrote a couple of years ago: “The end of insight.” The idea that humans should be able to understand the world around them is a relatively recent concept. “From my point of view as a mathematician, insight started with Isaac Newton,” Strogatz says. “So it’s like we’ve had 350 years of really good insight, where we’ve found that nature obeys beautiful mathematical patterns and we can understand them.”
Strogatz believes our window of insight is closing—that “we’re reaching our limitations.” In several fields, we seem to be approaching the limits of our intellectual abilities. “People talk about hundreds of billions of things in economics, in the brain, in genes,” Strogatz says. “Once you start talking about that kind of number, lots of interesting interactions occur, and that’s where the scientific frontier problems are—and we’re just not very good at thinking about those kinds of numbers.” And computers are.
Neither Strogatz nor Lipson have a date in mind for when humans will lose their mastery over science. Even as the robots get smarter and smarter, there will still be many human traits that science will depend on. For instance, taste—the ability to choose interesting, creative areas of science to investigate. But make no mistake: Our time is limited. “As thinking machines, they have a lot of advantages over us—this is obvious,” Strogatz says. “We’re not going to be the best players in town. I do think we’ll be put out of business. This is really going to happen.”
***
“This is really going to happen” is an appropriate epigram for this series. Over the last week I’ve covered a range of technologies, some more theoretical than others. It’s possible that some of the robots and automated systems I’ve covered will advance more slowly than I’m predicting, and a few technologies may fail completely. Eureqa is a good example of the wide range of possible outcomes. The program works for a small range of problems right now, and its inventors say it will get much more advanced in the future. But nobody knows for certain how good it will get and how quickly, and how it will change what scientists do every day.
But perhaps focusing on individual technologies is the wrong way to look at this story. If we zoom out and look at the pace at which computing power is increasing, the idea that machines will replace human workers becomes difficult to ignore. William Nordhaus, an economist at Yale, researches how “computer performance” has increased since the days of manual typewriters. He started with mechanical calculators of the 1800s, and then looked at how much better computers became as each new advance came along—from vacuum tubes to transistors to microchips. In 2007, he published his findings in the the Journal of Economic History. “Depending upon the standard used, computer performance has improved since manual computing by a factor between 1.7 trillion and 76 trillion,” Nordhaus wrote. This is a wide range, but even the most conservative number is staggering—in just 200 years, computers have made our species inhumanly good at calculating.
There are no signs that the rate of growth in computing power is slowing down. Moore’s law—the idea, roughly, that computers power doubles every couple of years—is still with us. The rise of ubiquitous networking and exponential increases in storage space guarantee that every year, machines will do more they did the year before. As Nordhaus says, computers “are a technology that has the potential for penetrating and fundamentally changing virtually every corner of economic life. At current rates of improvement, computers are approaching the complexity and computational capacity of the human brain. Perhaps computers will prove to be the ultimate outsourcer.”
I’d discount Nordaus’ “perhaps.” If you think us humans are secure in our jobs, you’d have to believe either a) that computers’ progress will slow or stop, or b) that humans will keep finding new jobs as machines take our old ones. Option A isn’t going to happen. And for most people, Option B is just a hope, because computers aren’t replacing one kind of human skill—they’re getting better at a wide range of skills that apply across many different jobs.
As I reported in this series, computers are improving their language and visual processing. They’re better than humans at remembering stuff and finding new connections, and they’re even making inroads into human creativity. At the moment, robots fall short in two main areas—they have a hard time manipulating objects in the physical world, and they’re not good at face-to-face conversation. But as computer power increases, the first of these problems will be solved. And the second—face-to-face conversation—might also be within their capacities in decades to come.
This series doesn’t address what happens next: What will humans do when computers have taken most of our jobs? How will we spend our time? How will we make money? How will society work when jobs are no longer the central activity of human existence?
I’ve ignored these deep questions because, at this point, very few people are thinking about them; they sound more like subjects that a stoner sci-fi geek would ponder than questions that serious people should spend time thinking about. But that’s got to change. Humanity is being eclipsed, and we need to figure out what to do about it. This is really going to happen.