A Tale of Four Algorithms
Each of these government algorithms is supposed to stop fraud and waste. Which works better—the one aimed at the poor or the rich?
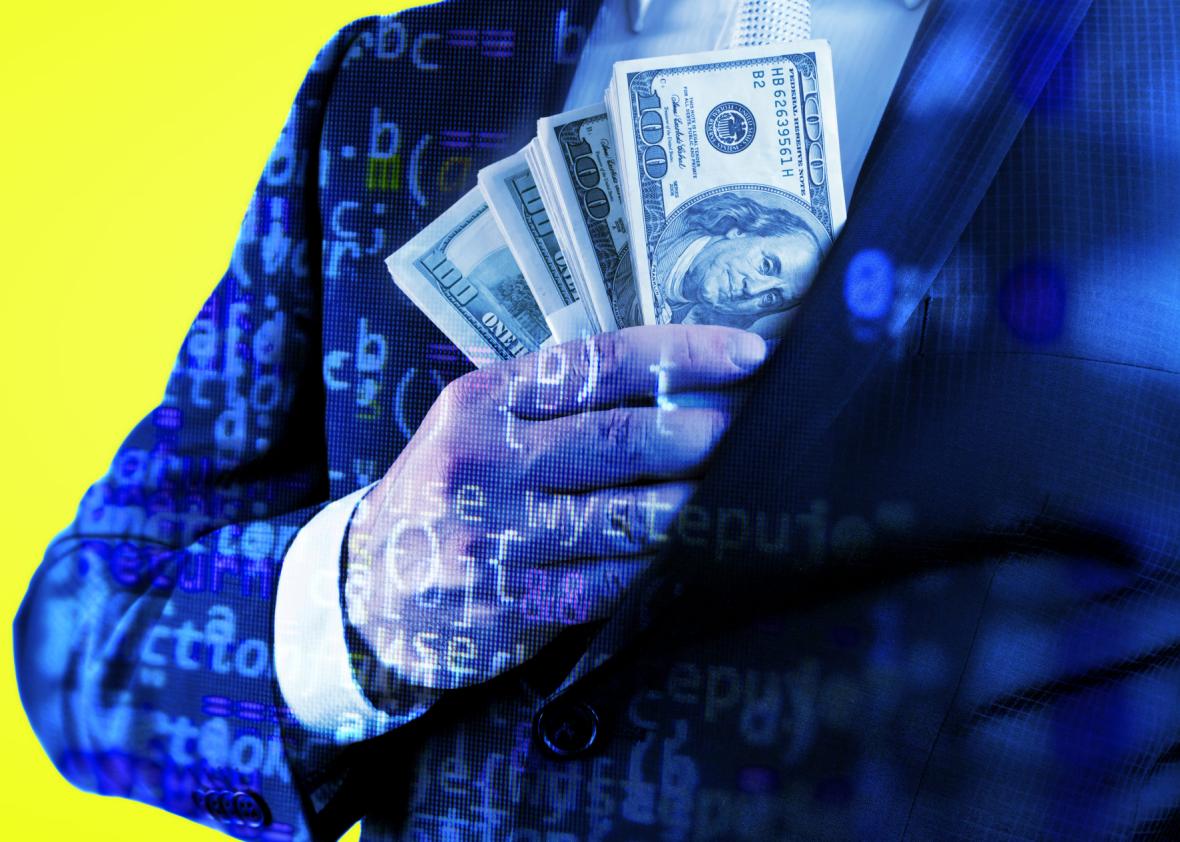
Illustration by Natalie Matthews-Ramo. Photos by iStock/Thinkstock.
Algorithms don’t just power search results and news feeds, shaping our experience of Google, Facebook, Amazon, Spotify, and Tinder. Algorithms are widely—and largely invisibly—integrated into American political life, policymaking, and program administration.
Algorithms can terminate your Medicaid benefits, exclude you from air travel, purge you from voter rolls, or predict if you are likely to commit a crime in the future. They make decisions about who has access to public services, who undergoes extra scrutiny, and where we target scarce resources.
But are all algorithms created equal? Does the kind of algorithm used by government agencies have anything to do with who it is aimed at?
Bias can enter algorithmic processes through many doors. Discriminatory data collection can mean extra scrutiny for whole communities, creating a feedback cycle of “garbage in, garbage out.” For example, much of the initial data that populated CalGang, an intelligence database used to target and track suspected gang members, was collected by the notorious Community Resources Against Street Hoodlums units of the LAPD, including in the scandal-ridden Rampart division. Algorithms can also mirror and reinforce entrenched cultural assumptions. For example, as Wendy Hui Kyong Chun has written, Googling “Asian + woman” a decade ago turned up more porn sites in the first 10 hits than a search for “pornography.”
But can automated policy decisions be class-biased? Let’s look at four algorithmic systems dedicated to one purpose—identifying and decreasing fraud, waste, and abuse in federal programs—each aimed at a different economic class. We’ll investigate the algorithms in terms of their effectiveness at protecting key American political values—efficacy, transparency, fairness, and accountability—and see which ones make the grade.
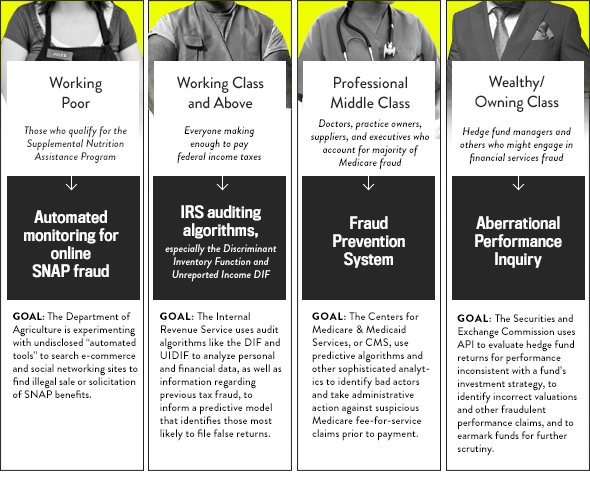
Below, I’ve scored each of the four policy algorithms on a scale of 1 to 5, 1 being very low and 5 being high. For example, if the algorithm didn’t beat a human being typing keywords into a search box, it scored very low efficacy (1). If it worked the way it was supposed to—if it identified mistakes in, say, a tax return but didn’t make much of a dent in the scope of tax fraud—it scored acceptable efficacy (3). If any of the algorithms both worked and substantially decreased fraud, waste, and abuse, it scored high efficacy (5). While the scoring is admittedly subjective, I set a pretty low bar for algorithmic success.
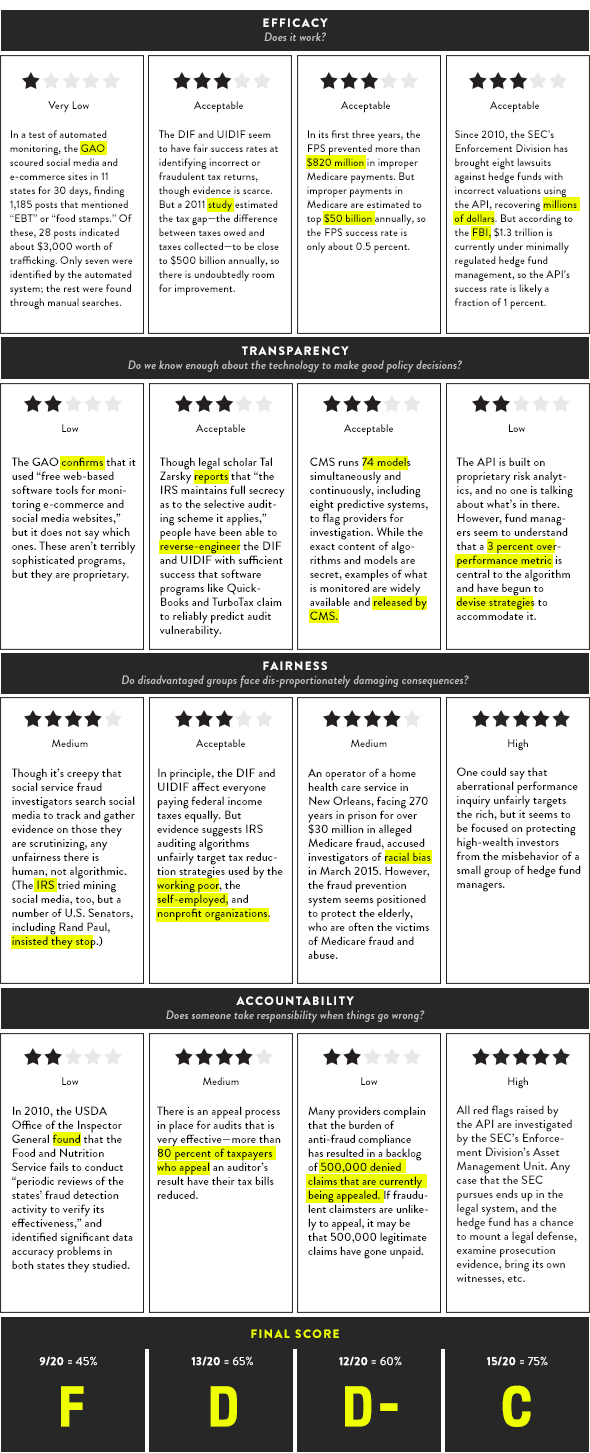
Of course this ad hoc survey is merely suggestive, not conclusive. But it indicates a reality that those of us who talk about data-driven policy rarely address: All algorithms are not created equal. Policymakers and programmers make inferences about their targets that get baked into the code of both legislation and high-tech administrative tools—that SNAP recipients are sneakier than other people and deserve less due process protection, for example.
Cultural assumptions about populations being modeled impact the model. Code is culture. None of the algorithms scored particularly well on all categories, but it is worth noting that the one targeting white-collar criminals received the highest score of all.
Unequally applied policy is not a problem that began in the age of computerization. The discretion of human decision-makers also creates grave injustices. But replacing biased individuals with biased systems may just speed up discrimination in public programs. Injustice is one thing we don’t want to make more efficient.
This article is part of the algorithm installment of Futurography, a series in which Future Tense introduces readers to the technologies that will define tomorrow. Each month from January through June 2016, we’ll choose a new technology and break it down. Read more from Futurography on algorithms:
- “What’s the Deal With Algorithms?”
- “Your Algorithms Cheat Sheet”
- “The Ethical Data Scientist”
- “How to Teach Yourself About Algorithms”
- “How to Hold Governments Accountable for the Algorithms They Use”
- “How Algorithms Are Changing the Way We Argue”
- “Algorithms Can Make Good Co-Workers”
- “Algorithms Aren’t Like Spock—They’re Like Capt. Kirk”
- “What Do We Not Want Algorithms to Do for Us?”
Future Tense is a collaboration among Arizona State University, New America, and Slate. To get the latest from Futurography in your inbox, sign up for the weekly Future Tense newsletter.