Safe?
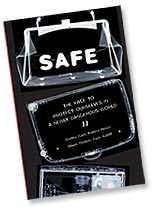
How will the technologies of the future help protect us against terrorism? A new book Safe: The Race To Protect Ourselves in a Newly Dangerous World examines innovative techniques for sniffing out attacks before they happen and for limiting damage if a strike does occur. In today's excerpt, the first of a three-part series, Martha Baer, Katrina Heron, Oliver Morton, and Evan Ratliff explain how to recognize potential terrorists with facial heat sensors and automated video cameras. Tomorrow's excerpt looks at a computer chip that could be the best weapon against bioterrorism. A final selection on Thursday considers a new technology that allows the government to root through citizens' private data without behaving like a police state.
What does a terrorist look like? Ask most Americans this question, and they will very likely say that a terrorist is a male, and perhaps that he is Arab, Muslim, young, and single. But examine the broader history of terrorist threats—from the Irish Republican Army to the Japanese Aum Shinrikyo cult to Oklahoma City bomber Timothy McVeigh to Ted Kaczynski to female Palestinian and Chechen suicide bombers—and that profile quickly falls apart. The common, self-evident thread among terrorists is not their ethnicity, age, or gender; it is their behavior. They stand out because of the brutal and sometimes suicidal acts of violence they commit—and the actions that precede that violence.
Much of our defense against such attacks typically focuses on the weapons terrorists might employ, from firearms, to explosives, to viruses, to nukes. Billions of dollars have been spent developing high-tech ways to seek out these physical representations of danger, with X-ray machines, explosive sniffers, and radiation detectors. But all such attacks, whether a hijacking or suicide bomb, consist of two elements: the weapon and the person who delivers it. The nature of the weapon changes, but the basic nature of the terrorist's intent does not. Is it possible, then, to uncloak terrorists based on their intentions, the subtleties of behavior that separate them from ordinary citizens?
A collection of researchers are working on exactly this question, employing both trained humans and machines to detect suspicious behavior. On the machine side, much of the research has focused on biometric technologies. The term "biometrics" refers generally to the application of statistical and mathematical models to biology, but it now also describes techniques that uniquely identify people based on biological traits. A few years ago, Ioannis Pavlidis, then a pattern-recognition expert at the high-tech conglomerate Honeywell, surveyed the biometrics landscape and quickly realized that all the burgeoning technologies fell into the same category: Whether fingerprint or face recognition, they were designed to find a distinguishing physical feature and match it to a person's identity in a database. That required already knowing who you were looking for—for example, making sure that the right person is accessing a secure door. The ultimate biometric, Pavlidis reasoned, would be not one that verified your identity but one that read your behavior without having to know it. "The idea was not, 'Tell me who you are,' " he says, "but, 'Tell me what you are about to do.' "
Pavlidis concluded that he might be able to measure stress by applying pattern recognition to a thermal-imaging system that would detect heat signatures produced by the body. Along with a renowned endocrinology researcher at the nearby Mayo Clinic named James Levine, he developed experiments to determine whether stress creates a unique thermal pattern in the face—one that could be detected with thermal-imaging cameras and software. Though temperature seems like an obvious indicator of stress, no effort had ever been made to detect and measure it at a distance.
During one early experiment, Pavlidis and Levine were in the lab, discussing what method to use to induce stress, a loud noise or a difficult math test. While they were debating this, a piece of ceramic tile they had brought along fell and crashed to the floor, startling their research subject. Suddenly, the monitors around the room lighted up, showing a quick but prominent flash of heat around the subject's eyes. They had found it: a thermal signal of the human "fight or flight" response. By using the temperature to infer things such as blood flow in the face, they could detect psychological conditions such as nervousness and fright, and possibly even deception.
The researchers are trying to perfect a system that would give questioners an additional tool to read their subjects' truthfulness. Unlike a traditional polygraph, which measures a combination of respiratory activity, fingertip sweat, and blood pressure, the thermal system doesn't require strapping in the subject. It can be conducted non-invasively and even without the subject's knowledge. Also, it doesn't require a specialist to interpret the results; the software simply spits out a score indicating the subject's truthfulness.
The downside of Pavlidis' system is that it only really works in a structured setting such as a visa interview—in a crowded airport, such technology would be rendered useless by the large numbers of nervous but innocent passengers it flagged. A nascent technology that could be used more widely is automated surveillance. Suspicious behavior is not confined to darting eyes, trembling hands, or heat signatures. When terrorists are probing their targets for information, or turn up somewhere they are not supposed to be, they advertise themselves as anomalies.
Video cameras are a natural—and often controversial—tool for trying to detect these macrobehaviors. The key to automated video surveillance is what computer-vision researchers call "blobology." First, the software takes pixels provided by the camera to create a statistical model of what is "normal" in the scene. Once normality has been established, the software looks for blobs: objects in the scene that don't fit that pattern. A person walking through the frame, a bird flying over, or a vehicle driving past would all be identified as blobs. When all the elements of a blob are established, the program determines what kind of object it is. Once the objects are classified—a human, a car, a piece of luggage—the algorithms can turn to identifying aberrant behaviors.
Take a hallway where all people are supposed to be traveling in the same direction.
Automated surveillance software can reliably detect when a person is moving the opposite way, and sound an alarm for a human operator. Major airports in the United States, including Dallas/Fort Worth and Salt Lake City, already use software to detect these kinds of extremely simple anomalous behaviors. A researcher in London has developed specialized algorithms for camera in the London Underground that pinpoint passengers who loiter too long on the platform or leave a bag behind. A scientist in San Diego developed crowd density rules that alerted authorities when certain stadium areas became too crowded during the Super Bowl.
The common thread among all the programs is that they look for violations of predefined rules. Imagine, for instance, that the same white van appears on consecutive days at an airport, driving slowly through the passenger pickup area—but each day at a different time. (Video surveillance tapes at Logan, in fact, reportedly showed hijacker Mohamed Atta doing just that, driving through the airport five times prior to Sept. 11.) To individual guards monitoring the area, the van would be a singular occurrence for their shift, not enough to represent an anomaly. But over multiple days, the reappearance of the van would constitute a pattern of unusual behavior—potentially someone surveilling the airport as a target.
The next step is to develop automated surveillance that doesn't need to be told in advance what constitutes a suspicious event. Because a camera can spend 24 hours a day, 365 days a year watching a scene, it could potentially be trained to catalog the range of normal behaviors in its field of vision—people usually walk a certain direction, vehicles typically stop for short periods of time. The system could then use that learning to develop its own rules for aberrant behaviors.
But besides the inevitable privacy questions, an entirely software-based video system—especially one designated to detect any activity it generally deems to be suspicious behavior—risks being plagued by false positives. Since the human ability to detect anomalies has not yet proved easy to replicate through technology, Boston's Logan Airport has decided to build a human-machine partnership. For starters, Logan officials have given out free cell phones to local clam diggers. At least for now, a guy looking for clams knows better than any video camera what constitutes an anomaly on the water.