While people may fear that artificial intelligence is getting too smart for our own good, researchers at Google have recently found that it still might not be clever enough to thwart human bad actors. In a study released in late December, researchers from Google designed a patch—which can be either a physical or digital image—that can trick machine learning into thinking an item is something else. In this case, the patch confounded machine learning into recognizing a banana as a toaster.
This isn’t the first “adversarial” technique that’s been used to fool A.I. (Remember these glasses? They obscured patterns used by advance facial recognition software enough to identify people, tricking it into thinking wearers looked like the opposite gender or even famous celebrities.) But the previous research in this area focused on full image manipulation and imperceptible changes that would need to be adjusted for each new attack. Google’s patch, by contrast, is “scene independent” and has a universal application. So, in theory, you could use the same patch to manipulate an image of a banana, a weapon, or something else, in any location.
What makes the finding even more formidable is researchers have found it effective in both “white box” settings (where researchers have access to the A.I.’s underlying algorithms) and “black box” settings (where they don’t.). Combine those two and you could get, say, bad actors using a sticker to manipulate security software into thinking a gun is a toaster. That’s quite scary, especially when humans actors are being used less and less to validate A.I. judgments. “Even if humans are able to notice these patches, they may not understand the intent of the patch and instead view it as a form of art,” researchers point out in the paper
The paper claims that the A.I. will identify an object as the faux toaster IRL with 99 percent confidence. You can see a Google researcher testing the physical patch against a banana here:
An A.I. skeptic, I took it upon myself to test this magic sticker using potential threats found at my desk: scissors and a pen. To do this, I downloaded Demitasse, an app the Google researchers recommend in their paper for at-home use. To test the app, I tried to identify both objects independent of any manipulations. It failed to identify the scissors at all. Out of roughly 10 trials, it only ranked “ballpoint” pen as the right object for my pen once.
I had slightly more luck with kitchen items. I was able to get the app to recognize both a banana and a printed picture of a toaster.
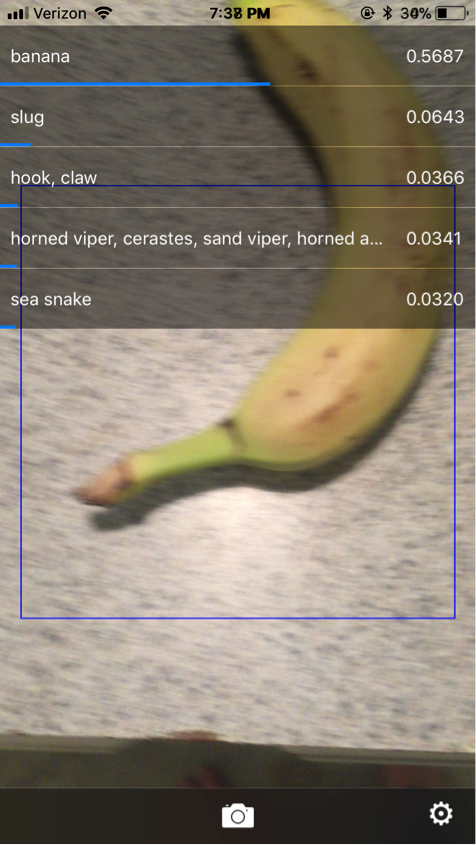
Screenshot from Demitasse
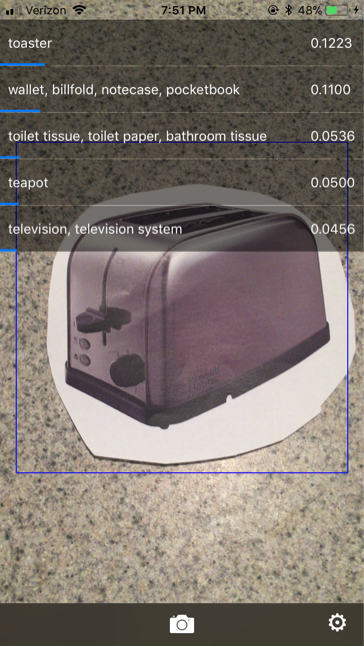
Screenshot from Demitasse
But when I combined the “adversarial” patch (which basically looks like a toaster melted in a rainbow) with my banana, the results were less spectacular:
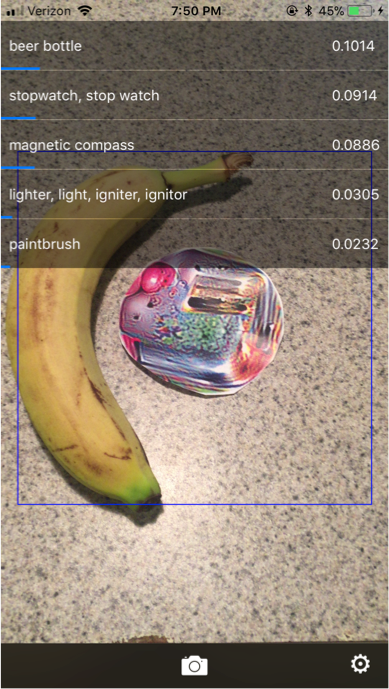
Screenshot from Demitasse
The app didn’t suggest the classifier output as a toaster or a banana—maybe not a big surprise given its struggle to identify either to begin with. Of course, there are a lot of things that could have gone wrong in my experiment. It involved an iPhone app (that kept crashing), a piece of paper, and a less than perfect-looking banana. The patch also isn’t optimized for printability, according to the paper. Tricking the app into thinking my scissors were a toaster likely also failed because the patch is less effective in universal applications unless it’s resized. That, and the fact that the app clearly wasn’t great.
Google’s menacing toaster Shrinky Dink shows that as much as we love to focus on its successes, imperfections are inevitable when it comes to mass utilization of machine learning.